Version 0.6-10 of NIMBLE released
We’ve released the newest version of NIMBLE on CRAN and on our website. Version 0.6-10 primarily contains updates to the NIMBLE internals that may speed up building and compilation of models and algorithms, as well as a few bug fixes.
Changes include:
- some steps of model and algorithm building and compilation are faster;
- compiled execution with multivariate distributions or function arguments may be faster;
- data can now be provided as a numeric data frame rather than a matrix;
- to run WAIC, a user now must set ‘enableWAIC’ to TRUE, either in NIMBLE’s options or as an argument to buildMCMC();
- if ‘enableWAIC’ is TRUE, buildMCMC() will now check to make sure that the nodes monitored by the MCMC algorithm will lead to a valid WAIC calculation; and
- the use of identityMatrix() is deprecated in favor of diag().
Please see the NEWS file in the installed package for more details
NIMBLE webinar Friday April 13
While analysts can use NIMBLE as a drop-in replacement for WinBUGS or JAGS, NIMBLE provides greatly enhanced functionality in a number of ways. The webinar will first show how to specify a hierarchical statistical model using BUGS syntax (including user-defined function and distributions) and fit that model using MCMC (including user customization for better performance). We will demonstrate the use of NIMBLE for biostatistical methods such as semiparametric random effects models and clustering models. We will close with a discussion of how to use the system to write algorithms for use with hierarchical models, including building and disseminating your own methods.
Adjunct Professor, Statistical Computing Consultant
Department of Statistics, University of California, Berkeley
Version 0.6-9 of NIMBLE released
We’ve released the newest version of NIMBLE on CRAN and on our website. Version 0.6-9 is primarily a maintenance release with various bug fixes and fixes for CRAN packaging issues.
New features include:
- dimensions in a model will now be determined from either ‘inits’ or ‘data’ if not otherwise available;
- one can now specify “nBootReps = NA” in the runCrossValidate() function, which will prevent the Monte Carlo error from being calculated;
- runCrossValidate() now returns the averaged loss over all k folds, instead of the summed loss;
- We’ve added the besselK function to the NIMBLE language;
- and a variety of bug fixes.
Please see the NEWS file in the installed package for more details
NIMBLE workshop in Switzerland, 23-25 April
There will be a three-day NIMBLE workshop in Sempach, Switzerland, 23-25 April, hosted at the Swiss Ornithological Institute. More information can be found here: http://www.phidot.org/forum/viewtopic.php?f=8&t=3586. Examples will be oriented towards ecological applications, but otherwise the workshop content will be general.
NIMBLE has a post-doc or software developer position open
The NIMBLE statistical software project at the University of California, Berkeley is looking for a post-doc or statistical software developer. NIMBLE is a tool for writing hierarchical statistical models and algorithms from R, with compilation via code-generated C++. Major methods currently include MCMC and sequential Monte Carlo, which users can customize and extend. More information can be found at https://R-nimble.org. Currently we seek someone with experience in computational statistical methods such as MCMC and excellent software development skills in R and C++. This could be someone with a Ph.D. in Statistics, Computer Science, or an applied statistical field in which they have done relevant work. Alternatively it could be someone with relevant experience in computational statistics and software engineering. The scope of work can include both core development of NIMBLE and development and application of innovative methods using NIMBLE, with specific focus depending on the background of the successful candidate. Applicants must have either a Ph.D. in a relevant field or have a proven record of relevant work. Please send cover letter, CV, and the names and contact information for three references to nimble.stats@gmail.com. Applications will be considered on a rolling basis starting 30 January, 2018.
Version 0.6-8 of NIMBLE released
We’ve released the newest version of NIMBLE on CRAN and on our website a week ago. Version 0.6-8 has a few new features, and more are on the way in the next few months.
New features include:
- the proper Gaussian CAR (conditional autoregressive) model can now be used in BUGS code as dcar_proper, which behaves similarly to BUGS’ car.proper distribution;
- a new nimbleMCMC function that provides one-line invocation of NIMBLE’s MCMC engine, akin to usage of JAGS and WinBUGS through R;
- a new runCrossValidate function that will conduct k-fold cross-validation of NIMBLE models fit by MCMC;
- dynamic indexing in BUGS code is now allowed by default;
- and a variety of bug fixes and efficiency improvements.
Please see the NEWS file in the installed package for more details.
Version 0.6-6 of NIMBLE released!
We’ve just released the newest version of NIMBLE on CRAN and on our website. Version 0.6-6 has some important new features, and more are on the way in the next few months.
Эти артефакты не имели практической или коммерческой ценности. На самом деле их продажа была строго запрещена обычаем. А так как предметы всегда находились в движении, их владельцы редко носили их. Тем не менее, массимы совершали модные подарки подруге долгие путешествия, чтобы обменять их, рискуя жизнью и здоровьем, когда они путешествовали по коварным водам Тихого океана на своих шатких каноэ.
New features include:
- dynamic indexes are now allowed in BUGS code — indexes of a variable no longer need to be constants but can be other nodes or functions of other nodes; for this release this is a beta feature that needs to be enabled with nimbleOptions(allowDynamicIndexing = TRUE);
- the intrinsic Gaussian CAR (conditional autoregressive) model can now be used in BUGS code as dcar_normal, which behaves similarly to BUGS’ car.normal distribution;
- optim is now part of the NIMBLE language and can be used in nimbleFunctions;
- it is possible to call out to external compiled code or back to R functions from a nimbleFunction using nimbleExternalCall() and nimbleRcall() (this is an experimental feature);
- the WAIC model selection criterion can be calculated using the calculateWAIC() method for MCMC objects;
- the bootstrap and auxiliary particle filters can now return their ESS values;
- and a variety of bug fixes.
Please see the NEWS file in the installed package for more details.
Finally, we’re deep in the midst of development work to enable automatic differentiation, Tensorflow as an alternative back-end computational engine, additional spatial models, and Bayesian nonparametrics.
Version 0.6-5 of NIMBLE released!
We’ve just released the newest version of NIMBLE on CRAN and on our website. Version 0.6-5 is mostly devoted to bug fixes and packaging fixes for CRAN, but there is some new functionality:
- addition of the functions c(), seq(), rep(), `:`, diag() for use in BUGS code;
- addition of two improper distributions (dflat and dhalfflat) as well as the inverse-Wishart distribution;
- the ability to estimate the asymptotic covariance of the estimates in NIMBLE’s MCEM algorithm;
- the ability to use nimbleLists in any nimbleFunction, newly including nimbleFunctions without setup code;
- and a variety of bug fixes and better error trapping.
Please see the NEWS file in the installed package for more details.
Better block sampling in MCMC with the Automated Factor Slice Sampler
One nice feature of NIMBLE’s MCMC system is that a user can easily write new samplers from R, combine them with NIMBLE’s samplers, and have them automatically compiled to C++ via the NIMBLE compiler. We’ve observed that block sampling using a simple adaptive multivariate random walk Metropolis-Hastings sampler doesn’t always work well in practice, so we decided to implement the Automated Factor Slice sampler (AFSS) of Tibbits, Groendyke, Haran, and Liechty (2014)
and see how it does on a (somewhat artificial) example with severe posterior correlation problems.
Roughly speaking, the AFSS works by conducting univariate slice sampling in directions determined by the eigenvectors of the marginal posterior covariance matrix for blocks of parameters in a model. So far, we’ve found the AFSS often outperforms random walk block sampling. To compare performance, we look at MCMC efficiency, which we define for each parameter as effective sample size (ESS) divided by computation time. We define overall MCMC efficiency as the minimum MCMC efficiency of all the parameters, because one needs all parameters to be well mixed.
We’ll demonstrate the performance of the AFSS on the correlated state space model described in Turek, de
.
Valpine, Paciorek, Anderson-Bergman, and others (2017)
Model Creation
Assume is the latent state and
is the observation at time
for
. We define the state space model as
for , with initial states
and prior distributions
where denotes a normal distribution with mean
and standard deviation
.
A file named model_SSMcorrelated.RData
with the BUGS model code, data, constants, and initial values for our model can be downloaded here.
## load the nimble library and set seed library('nimble') set.seed(1) load('model_SSMcorrelated.RData') ## build and compile the model stateSpaceModel <- nimbleModel(code = code, data = data, constants = constants, inits = inits, check = FALSE) C_stateSpaceModel <- compileNimble(stateSpaceModel)
Comparing two MCMC Samplers
We next compare the performance of two MCMC samplers on the state space model described above. The first sampler we consider is NIMBLE’s RW_block
sampler, a Metropolis-Hastings sampler with a multivariate normal proposal distribution. This sampler has an adaptive routine that modifies the proposal covariance to look like the empirical covariance of the posterior samples of the parameters. However, as we shall see below, this proposal covariance adaptation does not lead to efficient sampling for our state space model.
We first build and compile the MCMC algorithm.
RW_mcmcConfig <- configureMCMC(stateSpaceModel) RW_mcmcConfig$removeSamplers(c('a', 'b', 'sigOE', 'sigPN')) RW_mcmcConfig$addSampler(target = c('a', 'b', 'sigOE', 'sigPN'), type = 'RW_block') RW_mcmc <- buildMCMC(RW_mcmcConfig) C_RW_mcmc <- compileNimble(RW_mcmc, project = stateSpaceModel)
We next run the compiled MCMC algorithm for 10,000 iterations, recording the overall MCMC efficiency from the posterior output. The overall efficiency here is defined as , where ESS denotes the effective sample size, and
the total run-time of the sampling algorithm. The minimum is taken over all parameters that were sampled. We repeat this process 5 times to get a very rough idea of the average minimum efficiency for this combination of model and sampler.
RW_minEfficiency <- numeric(5) for(i in 1:5){ runTime <- system.time(C_RW_mcmc$run(50000, progressBar = FALSE))['elapsed'] RW_mcmcOutput <- as.mcmc(as.matrix(C_RW_mcmc$mvSamples)) RW_minEfficiency[i] <- min(effectiveSize(RW_mcmcOutput)/runTime) } summary(RW_minEfficiency)
## Min. 1st Qu. Median Mean 3rd Qu. Max. ## 0.3323 0.4800 0.5505 0.7567 0.7341 1.6869
Examining a trace plot of the output below, we see that the $a$ and $b$ parameters are mixing especially poorly.
plot(RW_mcmcOutput, density = FALSE)
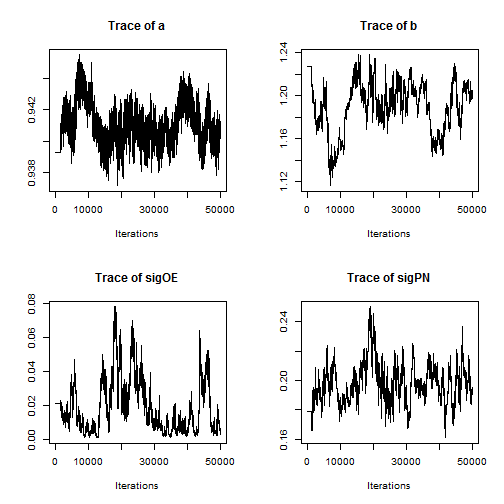
Plotting the posterior samples of against those of
reveals a strong negative correlation. This presents a problem for the Metropolis-Hastings sampler — we have found that adaptive algorithms used to tune the proposal covariance are often slow to reach a covariance that performs well for blocks of strongly correlated parameters.
plot.default(RW_mcmcOutput[,'a'], RW_mcmcOutput[,'b'])
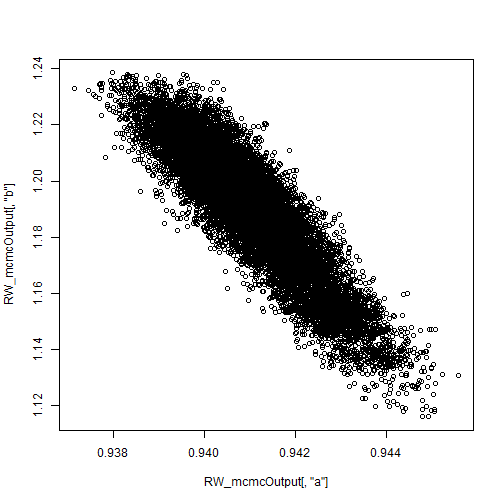
cor(RW_mcmcOutput[,'a'], RW_mcmcOutput[,'b'])
## [1] -0.9201277
In such situations with strong posterior correlation, we’ve found the AFSS to often run much more efficiently, so we next build and compile an MCMC algorithm using the AFSS sampler. Our hope is that the AFSS sampler will be better able to to produce efficient samples in the face of high posterior correlation.
AFSS_mcmcConfig <- configureMCMC(stateSpaceModel) AFSS_mcmcConfig$removeSamplers(c('a', 'b', 'sigOE', 'sigPN')) AFSS_mcmcConfig$addSampler(target = c('a', 'b', 'sigOE', 'sigPN'), type = 'AF_slice') AFSS_mcmc<- buildMCMC(AFSS_mcmcConfig) C_AFSS_mcmc <- compileNimble(AFSS_mcmc, project = stateSpaceModel, resetFunctions = TRUE)
We again run the AFSS MCMC algorithm 5 times, each with 10,000 MCMC iterations.
AFSS_minEfficiency <- numeric(5) for(i in 1:5){ runTime <- system.time(C_AFSS_mcmc$run(50000, progressBar = FALSE))['elapsed'] AFSS_mcmcOutput <- as.mcmc(as.matrix(C_AFSS_mcmc$mvSamples)) AFSS_minEfficiency[i] <- min(effectiveSize(AFSS_mcmcOutput)/runTime) } summary(AFSS_minEfficiency)
## Min. 1st Qu. Median Mean 3rd Qu. Max. ## 9.467 9.686 10.549 10.889 10.724 14.020
Note that the minimum overall efficiency of the AFSS sampler is approximately 28
times that of the RW_block
sampler. Additionally, trace plots from the output of the AFSS sampler show that the and
parameters are mixing much more effectively than they were under the
RW_block
sampler.
plot(AFSS_mcmcOutput, density = FALSE)
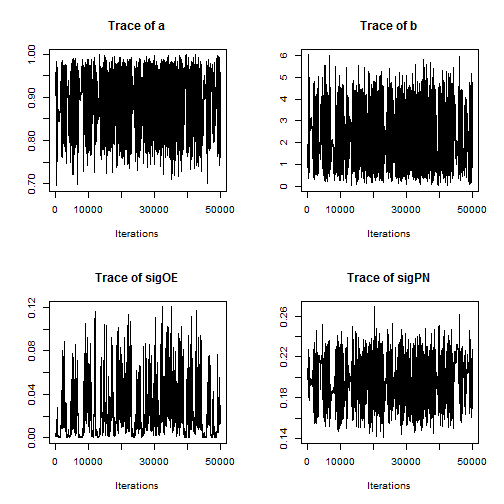
Tibbits, M. M, C. Groendyke, M. Haran, et al.
(2014).
“Automated factor slice sampling”.
In: Journal of Computational and Graphical Statistics 23.2, pp. 543–563.
Turek, D, P. de
Valpine, C. J. Paciorek, et al.
(2017).
“Automated parameter blocking for efficient Markov chain Monte Carlo sampling”.
In: Bayesian Analysis 12.2, pp. 465–490.
Version 0.6-4 of NIMBLE released!
We’ve just released the newest version of NIMBLE on CRAN and on our website. Version 0.6-4 has a bunch of new functionality for writing your own algorithms (using a natural R-like syntax) that can operate on user-provided models, specified using BUGS syntax. It also enhances the functionality of our built-in MCMC and other algorithms.
- addition of the functions c(), seq(), rep(), `:`, diag(), dim(), and which() for use in the NIMBLE language (i.e., run code) — usage generally mimics usage in R;
- a complete reorganization of the User Manual, with the goal of clarifying how one can write nimbleFunctions to program with models;
- addition of the adaptive factor slice sampler, which can improve MCMC sampling for correlated blocks of parameters;
- addition of a new sampler that can handle non-conjugate Dirichlet settings;
- addition of a nimbleList data structure that behaves like R lists for use in nimbleFunctions;
- addition of eigendecomposition and SVD functions for use in the NIMBLE language;
- additional flexibility in providing initial values for numeric(), logical(), integer(), matrix(), and array();
- logical vectors and operators can now be used in the NIMBLE language;
- indexing of vectors and matrices can now use arbitrary numeric and logical vectors;
- one can now index a vector of node names provided to values(), and more general indexing of node names in calculate(), simulate(), calculateDiff() and getLogProb();
- addition of the inverse-gamma distribution;
- use of recycling for distribution functions used in the NIMBLE language;
- enhanced MCMC configuration functionality;
- users can specify a user-defined BUGS distribution by simply providing a user-defined ‘d’ function without an ‘r’ function for use when an algorithm doesn’t need the ‘r’ function;
- and a variety of bug fixes, speedups, and better error trapping and checking.
Please see the NEWS file in the installed package for more details.