NIMBLE is hiring a programmer
This position includes work to harness parallel processing and automatic differentiation, to generate interfaces with other languages such as Python, to improve NIMBLE’s scope and efficiency for large statistical models, and to build other new features into NIMBLE.
The work will involve programming in R and C++, primarily designing and implementing software involving automated generation of C++ code for class and function definitions, parallel computing, use of external libraries for automatic differentiation and linear algebra, statistical algorithms and related problems. The position will also involve writing documentation and following good open-source software practices.
See here to apply.
Building Particle Filters and Particle MCMC in NIMBLE
nimble
‘s Particle Filtering AlgorithmsThis example shows how to construct and conduct inference on a state space model using particle filtering algorithms. nimble
currently has versions of the bootstrap filter, the auxiliary particle filter, the ensemble Kalman filter, and the Liu and West filter implemented. Additionally, particle MCMC samplers are available and can be specified for both univariate and multivariate parameters.
Model Creation
Assume is the latent state and
is the observation at time
for
. We define our state space model as
with initial states
and prior distributions
where denotes a normal distribution with mean
and standard deviation
, and
is a shifted, scaled
-distribution with center parameter
, scale parameter
, and
degrees of freedom.
We specify and build our state space model below, using time points:
## load the nimble library and set seed library('nimble') set.seed(1) ## define the model stateSpaceCode <- nimbleCode({ a ~ dunif(-0.9999, 0.9999) b ~ dnorm(0, sd = 1000) sigPN ~ dunif(1e-04, 1) sigOE ~ dunif(1e-04, 1) x[1] ~ dnorm(b/(1 - a), sd = sigPN/sqrt((1-a*a))) y[1] ~ dt(mu = x[1], sigma = sigOE, df = 5) for (i in 2:t) { x[i] ~ dnorm(a * x[i - 1] + b, sd = sigPN) y[i] ~ dt(mu = x[i], sigma = sigOE, df = 5) } }) ## define data, constants, and initial values data <- list( y = c(0.213, 1.025, 0.314, 0.521, 0.895, 1.74, 0.078, 0.474, 0.656, 0.802) ) constants <- list( t = 10 ) inits <- list( a = 0, b = .5, sigPN = .1, sigOE = .05 ) ## build the model stateSpaceModel <- nimbleModel(stateSpaceCode, data = data, constants = constants, inits = inits, check = FALSE)
## defining model...
## building model...
## setting data and initial values...
## running calculate on model (any error reports that follow may simply ## reflect missing values in model variables) ...
##
## checking model sizes and dimensions...
## note that missing values (NAs) or non-finite values were found in model ## variables: x, lifted_a_times_x_oBi_minus_1_cB_plus_b. This is not an error, ## but some or all variables may need to be initialized for certain algorithms ## to operate properly.
##
## model building finished.
Construct and run a bootstrap filter
We next construct a bootstrap filter to conduct inference on the latent states of our state space model. Note that the bootstrap filter, along with the auxiliary particle filter and the ensemble Kalman filter, treat the top-level parameters a, b, sigPN
, and sigOE
as fixed. Therefore, the bootstrap filter below will proceed as though a = 0, b = .5, sigPN = .1
, and sigOE = .05
, which are the initial values that were assigned to the top-level parameters.
The bootstrap filter takes as arguments the name of the model and the name of the latent state variable within the model. The filter can also take a control list that can be used to fine-tune the algorithm’s configuration.
## build bootstrap filter and compile model and filter bootstrapFilter <- buildBootstrapFilter(stateSpaceModel, nodes = 'x') compiledList <- compileNimble(stateSpaceModel, bootstrapFilter)
## compiling... this may take a minute. Use 'showCompilerOutput = TRUE' to see C++ compiler details.
## compilation finished.
## run compiled filter with 10,000 particles. ## note that the bootstrap filter returns an estimate of the log-likelihood of the model. compiledList$bootstrapFilter$run(10000)
## [1] -28.13009
Particle filtering algorithms in nimble
store weighted samples of the filtering distribution of the latent states in the mvSamples
modelValues object. Equally weighted samples are stored in the mvEWSamples
object. By default, nimble
only stores samples from the final time point.
## extract equally weighted posterior samples of x[10] and create a histogram posteriorSamples <- as.matrix(compiledList$bootstrapFilter$mvEWSamples) hist(posteriorSamples)
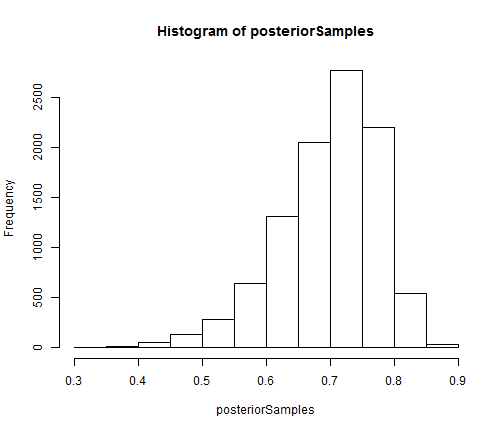
The auxiliary particle filter and ensemble Kalman filter can be constructed and run in the same manner as the bootstrap filter.
Conduct inference on top-level parameters using particle MCMC
Particle MCMC can be used to conduct inference on the posterior distribution of both the latent states and any top-level parameters of interest in a state space model. The particle marginal Metropolis-Hastings sampler can be specified to jointly sample the a, b, sigPN
, and sigOE
top level parameters within nimble
‘s MCMC framework as follows:
## create MCMC specification for the state space model stateSpaceMCMCconf <- configureMCMC(stateSpaceModel, nodes = NULL) ## add a block pMCMC sampler for a, b, sigPN, and sigOE stateSpaceMCMCconf$addSampler(target = c('a', 'b', 'sigPN', 'sigOE'), type = 'RW_PF_block', control = list(latents = 'x')) ## build and compile pMCMC sampler stateSpaceMCMC <- buildMCMC(stateSpaceMCMCconf) compiledList <- compileNimble(stateSpaceModel, stateSpaceMCMC, resetFunctions = TRUE)
## compiling... this may take a minute. Use 'showCompilerOutput = TRUE' to see C++ compiler details.
## compilation finished.
## run compiled sampler for 5000 iterations compiledList$stateSpaceMCMC$run(5000)
## |-------------|-------------|-------------|-------------| ## |-------------------------------------------------------|
## NULL
## create trace plots for each parameter library('coda')
par(mfrow = c(2,2)) posteriorSamps <- as.mcmc(as.matrix(compiledList$stateSpaceMCMC$mvSamples)) traceplot(posteriorSamps[,'a'], ylab = 'a') traceplot(posteriorSamps[,'b'], ylab = 'b') traceplot(posteriorSamps[,'sigPN'], ylab = 'sigPN') traceplot(posteriorSamps[,'sigOE'], ylab = 'sigOE')
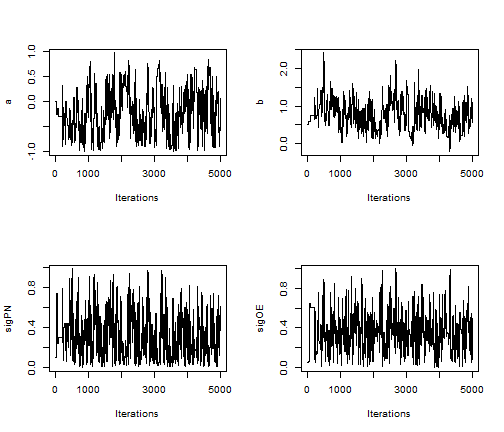
The above RW_PF_block
sampler uses a multivariate normal proposal distribution to sample vectors of top-level parameters. To sample a scalar top-level parameter, use the RW_PF
sampler instead.
Version 0.6-3 released.
Version 0.6-3 is a very minor release primarily intended to address some CRAN packaging issues that do not affect users. We also fixed a bug involving MCEM functionality and a bug that prevented use of the sd() and var() functions in BUGS code.
For most users, there is probably no need to upgrade from version 0.6-2.
Version 0.6-2 released!
Version 0.6-2 is a minor release with a variety of useful functionality for users.
Changes as of Version 0.6-2 include:
- user-defined distributions can be used in BUGS code without needing to call the registerDistributions() function (unless one wants to specify alternative parameterizations, distribution range or that the distribution is discrete),
- users can now specify the use of conjugate (Gibbs) samplers for nodes in a model,
- NIMBLE will now check the run code of nimbleFunctions for functions (in particular R functions) that are not part of the DSL and will not compile,
- added getBound() functionality to find the lower and upper bounds of a node either from R or in DSL code,
- added functionality to get distributional information about a node in a model or information about a distribution based on the name of the density function; these may be useful in setup code for algorithms,
- multinomial and categorical distributions now allow ‘probs’ arguments that do not sum to one (these will be internally normalized) and
- a variety of bug fixes.
Please see the NEWS file in the installed package for more details.
NIMBLE package for hierarchical modeling (MCMC and more) faster and more flexible in version 0.6-1
NIMBLE version 0.6-1 has been released on CRAN and at r-nimble.org.
NIMBLE is a system that allows you to:
- Write general hierarchical statistical models in BUGS code and create a corresponding model object to use in R.
- Build Markov chain Monte Carlo (MCMC), particle filters, Monte Carlo Expectation Maximization (MCEM), or write generic algorithms that can be applied to any model.
- Compile models and algorithms via problem-specific generated C++ that NIMBLE interfaces to R for you.
Most people associate BUGS with MCMC, but NIMBLE is about much more than that. It implements and extends the BUGS language as a flexible system for model declaration and lets you do what you want with the resulting models. Some of the cool things you can do with NIMBLE include:
- Extend BUGS with functions and distributions you write in R as nimbleFunctions, which will be automatically turned into C++ and compiled into your model.
- Program with models written in BUGS code: get and set values of variables, control model calculations, simulate new values, use different data sets in the same model, and more.
- Write your own MCMC samplers as nimbleFunctions and use them in combination with NIMBLE’s samplers.
- Write functions that use MCMC as one step of a larger algorithm.
- Use standard particle filter methods or write your own.
- Combine particle filters with MCMC as Particle MCMC methods.
- Write other kinds of model-generic algorithms as nimbleFunctions.
- Compile a subset of R’s math syntax to C++ automatically, without writing any C++ yourself.
Some early versions of NIMBLE were not on CRAN because NIMBLE’s system for on-the-fly compilation via generating and compiling C++ from R required some extra work for CRAN packaging, but now it’s there. Compared to earlier versions, the new version is faster and more flexible in a lot of ways. Building and compiling models and algorithms could sometimes get bogged down for large models, so we streamlined those steps quite a lot. We’ve generally increased the efficiency of C++ generated by the NIMBLE compiler. We’ve added functionality to what can be compiled to C++ from nimbleFunctions. And we’ve added a bunch of better error-trapping and informative messages, although there is still a good way to go on that. Give us a holler on the nimble-users list if you run into questions.
Version 0.5-1 of NIMBLE released!
Version 0.5-1 is officially a minor release, but it actually has quite a bit in it, in particular the addition/improvement of a number of our algorithms. In addition there are some more improvements in our speed in building and compiling models and algorithms.
Changes as of Version 0.5-1 include:
- the addition of a variety of sequential Monte Carlo (aka particle filtering) algorithms, including particle MCMC samplers for use within an MCMC,
- a greatly improved MCEM algorithm with an automated convergence and stopping criterion,
- new syntax for declaring multivariate variables in the NIMBLE DSL, namely numeric(), integer(), matrix(), and array(), with declare() now deprecated,
- addition of the multivariate-t distribution for use in BUGS and DSL code,
- a new binary MCMC sampler for discrete 0/1 nodes,
- addition of functionality to our random walk sampler to allow sampling on the log scale and use of reflection,
- more flexible use of forwardsolve(), backsolve(), and solve(), including use in BUGS code, and
- a variety of other items.
Please see the NEWS file in the source package.
NIMBLE: A new way to do MCMC (and more) from BUGS code in R
Yesterday we released version 0.5 of NIMBLE on our web site, r-nimble.org. (We’ll get it onto CRAN soon, but it has some special needs to work out.) NIMBLE tries to fill a gap in what R programmers and analysts can do with general hierarchical models. Packages like WinBUGS, OpenBUGS, JAGS and Stan provide a language for writing a model flexibly, and then they provide one flavor of MCMC. These have been workhorses of the Bayesian revolution, but they don’t provide much control over how the MCMC works (what samplers are used) or let one do anything else with the model (though Stan provides some additional fitting methods).
The idea of NIMBLE has been to provide a layer of programmability for algorithms that use models written in BUGS. We adopted BUGS as a model declaration language because these is so much BUGS code out there and so many books that use BUGS for teaching Bayesian statistics. Our implementation processes BUGS code in R and creates a model object that you can program with. For MCMC, we provide a default set of samplers, but these choices can be modified. It is easy to write your own sampler and add it to the MCMC. And it is easy to add new distributions and functions for use in BUGS code, something that hasn’t been possible (in any easy way) before. These features can allow big gains in MCMC efficiency.
MCMCs are heavily computational, so NIMBLE includes a compiler that generates C++ specific to a model and algorithm (MCMC samplers or otherwise), compiles it, loads it into R and gives you an interface to it. To be able to compile an algorithm, you need to write it as a nimbleFunction rather than a regular R function. nimbleFunctions can interact with model objects, and they can use a subset of R for math and flow-control. Among other things, the NIMBLE compiler automatically generates code for the Eigen C++ linear algebra library and manages all the necessary interfaces.
Actually, NIMBLE is not specific to MCMC or to Bayesian methods. You can write other algorithms to use whatever model you write in BUGS code. Here’s one simple example: in the past if you wanted to do a simulation study for a model written in BUGS code, you had to re-write the model in R just to simulate from it. With NIMBLE you can simulate from the model as written in BUGS and have complete control over what parts of the model you use. You can also query the model about how nodes are related so that you can make an algorithm adapt to what it finds in a model. We have a set of sequential Monte Carlo (particle filter) methods in development that we’ll release soon. But the idea is that NIMBLE provides a platform for others to develop and disseminate model-generic algorithms.
NIMBLE also extends BUGS in a bunch of ways that I won’t go into here. And it has one major limitation right now: it doesn’t handle models with stochastic indices, like latent class membership models.
Here is a toy example of what it looks like to set up and run an MCMC using NIMBLE.
library(nimble) myBUGScode <- nimbleCode({ mu ~ dnorm(0, sd = 100) ## uninformative prior sigma ~ dunif(0, 100) for(i in 1:10) y[i] ~ dnorm(mu, sd = sigma) }) myModel <- nimbleModel(myBUGScode)
myData <- rnorm(10, mean = 2, sd = 5) myModel$setData(list(y = myData)) myModel$setInits(list(mu = 0, sigma = 1)) myMCMC <- buildMCMC(myModel) compiled <- compileNimble(myModel, myMCMC) compiled$myMCMC$run(10000)
samples <- as.matrix(compiled$myMCMC$mvSamples) plot(density(samples[,'mu']))
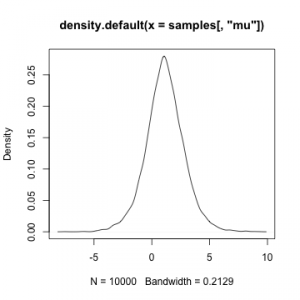
plot(density(samples[,'sigma']))
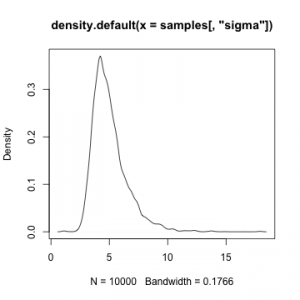
NIMBLE paper in Journal of Computational and Graphical Statistics
Our paper giving an overview on the rationale and design of NIMBLE has appeared online in accepted manuscript form at the Journal of Computational and Graphical Statistics. You can get it here.
Version 0.5 released!
We’ve just released the next major version of NIMBLE.
Changes include
- more efficient computations for conjugate sampling,
- additional automated checking of BUGS syntax to improve NIMBLE’s warning/error messages,
- new API functionality to allow the use of syntax such as model$calculate(), etc. (syntax such as calculate(model) still works),
- new API functionality for MCMC sampler specification,
- improvements in speed and memory use in building models,
- addition of forwardsolve, backsolve, and solve to the NIMBLE DSL, and
- a variety of other items.
More details in the NEWS file that accompanies the package.
We anticipate being on CRAN in coming weeks and a next release soon that will include a full suite of sequential Monte Carlo (i.e., particle filtering) algorithms.
Version 0.4-1 released!
We’ve just released version 0.4-1, a minor release that fixes some logistical issues and adds a bit of functionality to our MCMC engine.
Changes as of Version 0.4-1 include:
- added an elliptical slice sampler to the MCMC engine,
- fixed bug preventing use of nimbleFunctions in packages depending on NIMBLE, and
- reduced C++ compiler warnings on Windows during use of compileNimble.